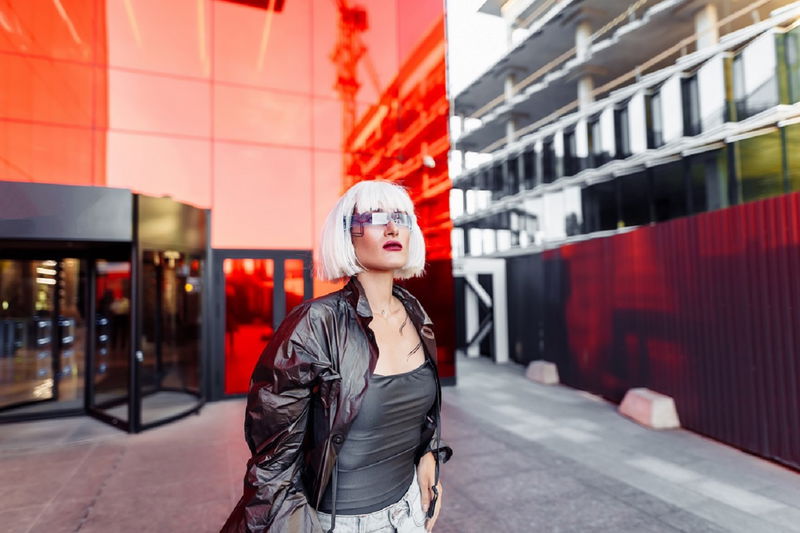
As data can constantly evolve, extracting value from it to innovate is vital in reaching wider audiences, offering more consumer-oriented services, and working faster and smarter as a business user.
Artificial intelligence and machine learning are enabling this trend by giving even old data newfound life driven by exponential data production/consumption growth.
Data Deluge: What It Is?
What is data deluge in data processing? A "data deluge" occurs when more data than can be effectively managed or contained is produced than can be managed or contained, leading to missed opportunities to develop conceptual and practical understandings, analyze facts for decision-making purposes, as well as make sound judgments. Every second, large volumes of information are produced.
Now, all we do online--interactions on social media platforms, web browsing and communication--produces massive volumes of data too large for current data processing legacy systems to handle. Furthermore, our ability to produce huge volumes of information continues to outstrip infrastructure necessary for conducting data-driven research projects.
Given the rapid expansion of digital data, delaying action makes sense, given its rate. Although organizing, rationalizing, and maintaining suitable long-term infrastructures for large amounts of information must begin immediately; an emerging economy's rapid development contributed heavily to an unprecedented data flood; but that was just the start; there's much more at play here than appears initially.
Strategies To Deal With Data Deluge
As digital technologies increase data production exponentially, an unprecedented level of information is generated. From every click and swipe online transaction - purchases or social media interactions - organizations need to balance opportunities against limits to access meaningful insights for decision-making purposes and navigate this data deluge successfully. Smart approaches with effective data analysis techniques must also be adopted.
1. Understanding Of Data Environment
Before embarking on any data analysis project, understanding the landscape is vitally important. There are three different kinds of data - semi-structured, unstructured and structured. While structured databases make for relatively straightforward analyses, unstructured text documents, images, or videos present more challenges due to lacking predetermined structures; semi-structured information bridges this divide by providing both flexibility and organization in equal measures.
2. Establish Goals And Key Measures
Setting clear goals is integral to successful data analysis. Institutions must outline exactly which queries or issues they wish to tackle through analysis. Well-outlined goals provide direction and provide structure for this process - such as optimizing marketing campaigns efforts, increasing customer experience or increasing operational efficiency. Defining key indicators also facilitates tracking progress against company goals more easily and ensures alignment. Employ internet research and data processing services for your company.
3. Collecting And Preparing Data
Data quality directly impacts insights gained through data analysis. Data can be collected from various sources - internal databases, outside providers and Internet of Things devices, among them - though due to issues like missing numbers, inconsistencies or outliers, raw data often needs preprocessing and cleansing before analysis can take place accurately. Cleaning, transformation and normalization tasks ensure accurate analyses.
Communicating and working effectively between development and operations teams within your company is an essential first step towards efficient operation. Although romantic in theory, reality often differs; developers and operations teams prioritize different things - Ops teams tend to prioritize effective data management strategies, while development teams prioritize product velocity and release cadence as priorities.
4. Selecting Appropriate Analytical Methods
After collecting data for analysis, the next step should be selecting suitable analytical methods. Deliberations upon which methods to employ should depend upon both its goals and the type of information being examined; predictive analytics offers insight into future outcomes based on past patterns, while descriptive analytics offers a deeper understanding of past data patterns and trends. Prescriptive analytics makes suggestions on how to enhance results through forecasted information.
One of the key steps towards making such plans a reality is the efficient utilization of information. Organizations must ensure metadata is comprehensive, accurate, and robust enough to facilitate cohesion among applications as well as rapidly identify data's purpose or business use case quickly and precisely allowing data categorization/separation efforts effectively.
5. Utilization Of Cutting-Edge Tools And Technologies
Technology innovations have rendered data analysis accessible and democratized for businesses of all sizes, providing advanced platforms and tools. There is now an abundance of tools that make analysis simpler, ranging from business intelligence (BI), data visualization tools and statistical software such as R and Python for simplifying this process; big data platforms provide large dataset processing capability while cloud computing technologies ensure large scale processing capability for large datasets.
But this brings us full circle to productive teamwork: Ops teams and developers must collaborate closely in communicating objectives and goals across teams in order to segregate data properly. Restricting communication to certain areas makes it impossible to locate or prioritize information within silos effectively; cultural change often provides more effective data management solutions than any technical approach can.
Related Article- Unlocking The Power Of Productivity: How To Find Top Virtual Assistant
6. Iterative Determination And Ongoing Enhancement
Data analysis should be considered an iterative process rather than a one-off activity for organizations to make effective use of. Through continuous tracking of key metrics and feedback loops, organizations are able to monitor performance, recognize emerging patterns, modify strategy accordingly and continuously advance decision-making procedures.
Data analytics is evolving with advances in technology. Automation and predictive modeling have become more accessible due to artificial intelligence (AI) and machine learning (ML). Meanwhile, massive quantities of sensor data produced by IoT provide insight into consumer behavior and real-time operations; decision-makers now find acquiring complex information easier due to improvements in data visualization techniques.
7. Accepting A Culture Driven By Data
Organizations need a culture that values data-driven decision-making at every level if they wish to realize the full potential of data analysis. This involves encouraging staff members to become data literate while investing in technological capabilities; workshops and training courses provide employees with essential skills needed for correctly analyzing data to produce actionable insights that can be implemented; furthermore, embedding analytics into business plans, processes, and prioritizing initiatives requires leadership support for data initiatives.
Networking and information exchange with peers and professionals are best accomplished via industry forums, conferences, or online communities. Partnerships between research groups and academic institutions foster creativity as well as drive new technologies and methods of data analysis; organizations may stay at the forefront by cultivating an environment conducive to cooperation and learning that continues over time.
8. Overcoming Obstacles And Difficulties
Data analytics offers immense promise to businesses; however, its implementation requires them to remain mindful of potential pitfalls and perils that lie in wait. Business leaders must recognize how complex data integration may become when managing multiple sources and complying with regulations; data security/privacy issues become ever more pressing. To mitigate such potential dangers it's essential for business goals that data analyses take proactive measures against those obstacles/perils encountered from data.
Preserving privacy, providing open data collection and application processes, and eliminating prejudices that support discrimination or inequality are essential components of responsible data use. Trade associations and regulatory agencies publish frameworks and ethical guidelines which serve as the cornerstone for ethical data practices; businesses may increase stakeholder trust while decreasing risks related to misuse by giving ethics in data analysis top priority.
How Can The Data Deluge Be Managed?
How do you overcome data deluge? Implementing an extensive modern data management system plan allows huge volumes of information to provide new valuable insights and drive improved business processes; otherwise, it becomes overwhelming and unmanageable, leaving businesses scrambling for ways to use or dispose of it all.
- Steer clear of data hoarding: conclusions drawn from large sets of unrelated or irrelevant information can often prove highly incorrect.
- Errors in data: Errors become clear once it becomes evident why and how you are gathering the information, along with why holding onto irrelevant information could ultimately harm the company.
- Removing silos: Silos must be broken apart so you can understand their true impact on your data ecosystem as a whole. They hinder companies from extracting maximum value from their stored information while creating costlier duplicate copies that could compromise employee security and lead to costly data duplication issues.
- Create an information-led culture: information must be integrated, validated and documented before being made accessible to appropriate parties - or more crucially equipment - where applicable.
- Select appropriate use cases and prepare data: A primary objective behind most firms' massive data collections efforts is their desire to utilize artificial intelligence (AI) analysis of collected information for improved business decisions. Business objectives equipped with strong capabilities for data collecting, storage and analysis stand to benefit more.
Data has become the cornerstone of company and business intelligence. Today, we work in environments with vast quantities of daily generated or collected information; IT must manage this load without interrupting business activities too severely.
Conclusion
Effective data analysis has become essential for businesses seeking to thrive in today's increasingly data-rich environment, not an optional extra. Organizations can tap the full potential of their data assets while confidently handling a deluge of information by adopting ethical considerations, utilizing cutting-edge tools and technologies, and adopting strategic methods. Leveraging insights derived from this vast repository will only become increasingly vital as data continues to shape our world today and tomorrow.